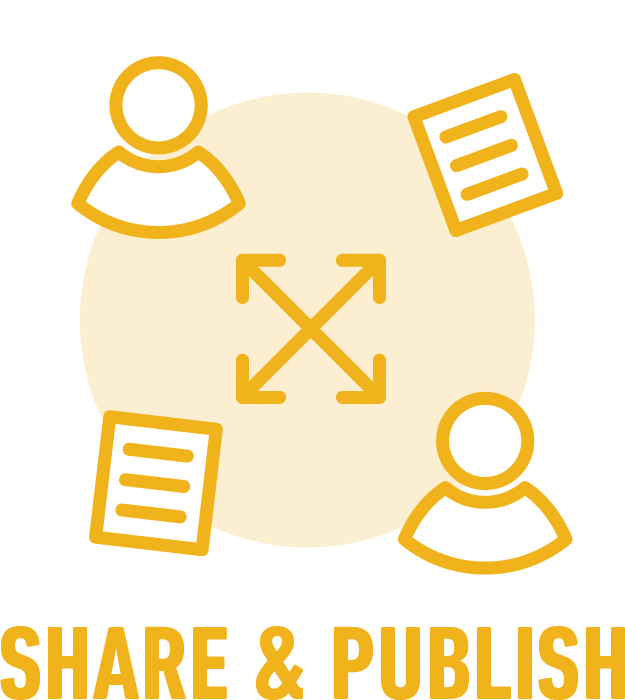
What?
Publishing research data is the first step towards data sharing. The collection and re-use of relevant, related (open) datasets supports an efficient continuum of relevant future research. Academics and researchers are encouraged or mandated, to share and publish research findings (raw or processed) in an open data repository making your data FAIR- Findable, Accessible, Interoperable and Reusable .
Why?
Publishing of data according to the FAIR guiding principles enables, amongst other things, maximum impact. For maximum impact, both research outputs and research data should be publicly available on an open-access platform. Making research objects and research data available ‘as open as possible and as closed as necessary’ enables ethical research integrity. DLS supports publishing of research output, because sharing data contributes to science and broadens UCT’s global research footprint as it:
- encourages enquiry and debates
- promotes innovation and new ways of using data
- leads to increased collaborations between data creators and users
- increases transparency and accountability
- enables scrutiny and validation of research output
- encourages the improvement of research methods
- reduces the time and cost spent on duplicating data collection
- increases the impact and visibility of research
- advances your academic ranking through increased coverage and citations
- contributes material and content for teaching and learning
How?
You can share data on UCT's institutional repository ZivaHub . To get started, consult our page for an introduction. For further assistance with using the ZivaHub platform, powered by Figshare for Institutions, consult the rest of our guides . Before publishing any research output on ZivaHub, you are required to read and accept the UCT terms of data deposit. Guidance on publishing and sharing sensitive data here .
FAIR open data
If you would like to engage more deeply with Open Science through open data publication, the FAIR principles (Findable, Accessible, Interoperable and Reusable) are widely regarded as the key schema for analysing the openness of a dataset. The FAIR principles were drafted at a Lorentz Center workshop in the Netherlands in 2015, and have since been taken up by major organisations such as FORCE11 , National Institutes of Health (NIH) and the European Commission.
The FAIR principles are a way of determining the openness of a particular dataset, as well as providing advice on how to make your data more open. The Australian Research Data Commons has created a FAIR data self-assessment tool you can use to determine the openness of your data. You can also look at the brief guidelines below to see how to make your data increasingly FAIR,
-
How do I make my data Findable?
-
How do I make my data Accessible?
Clearly indicate under what conditions the data may be reused. There are good reasons not to share certain kinds of data, such as data owned by commercial partners, confidential data, or disclosive data about at-risk populations. This may be fully open (such as under a Creative Commons Attribution-Only) licence, or with specific usage constraints. UCT's DataFirst repository has a number of access conditions for different levels of sensitivity, while ZivaHub has the option to make the metadata open while keeping the data files confidential.
-
How do I make my data Interoperable?
For data interoperability disciplinary or community data standards, formats and standardised vocabulary can be used for data description. Metadata should adhere to disciplinary standards, optionally linking out to related information using identifiers.
-
How do I make my data Reusable?
- To make the data findable, ascribe the dataset with metadata to assist users understand the data. Using discipline-specific metadata further enhances its reusability.
- Ensure that the chosen repository has machine-readable licences, which is increasingly standard. Both ZivaHub and DataFirst provide machine-readable licences.
5-Star Open Data
Another schema for judging the openness of a particular set of data is the 5-Star Open Data model developed Tim Berners-Lee. The 5-Star Open Data model describes data shared online according to how open it is, with the following ratings:
1-Star | the data is online and under an open licence. |
2-Star | the data is online and under an open licence, and is in a structured form (i.e. MS Excel format, rather than a picture of a table). |
3-Star | the data is online and under an open licence, and is in a structured form in a non-proprietary format (i.e. .csv instead of .xlsx). |
4-Star | the data is online and under an open licence, in a structured form in a non-proprietary format, using URIs. |
5-Star | the data is online and under an open licence, in a structured form in a non-proprietary format, using URIs, and links to other data to provide context. |
The 5-Star model is graphically displayed below:
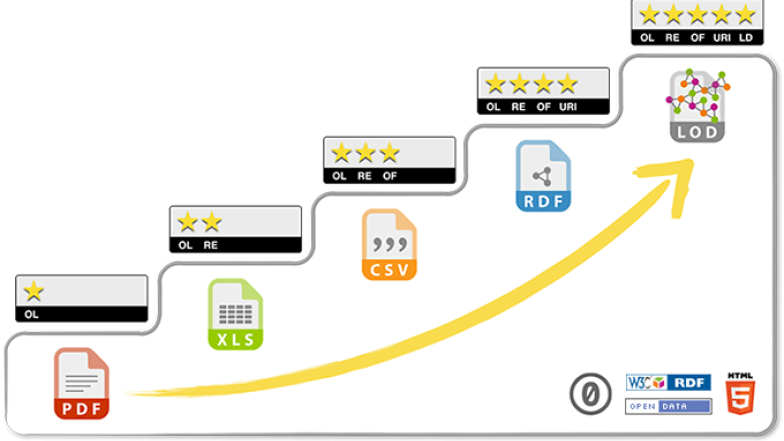
The 5-Star Open Data model is geared towards quantitative data, and reaching the 4-Star and 5-Star levels requires specific kinds of data and data expertise that may not be possible in all projects.
For more information on the model, please visit the 5-Star website .
Further reading
If you would like to read further into the field of research data management (RDM), a number of institutions, consortia and other high-level bodies have created guidance on how to implement RDM, listed below:
- ANDS (Australian National Data Service): Data management overview
- DCC (Digital Curation Centre): Making the Case for Research Data Management
- JISC (Joint Information Systems Committee): Managing research data in your institution
- OCLC (Online Computer Library Center): The Realities of Research Data Management
Explore the RDM life cycle
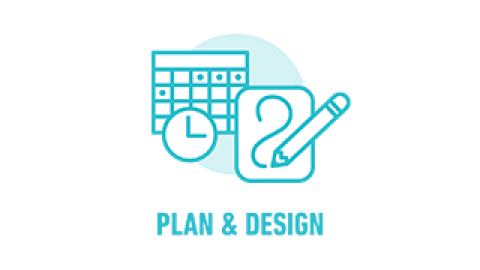
Plan & Design
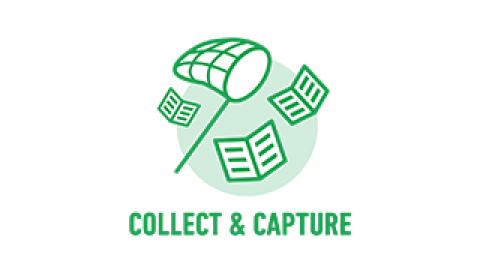
Collect & Capture
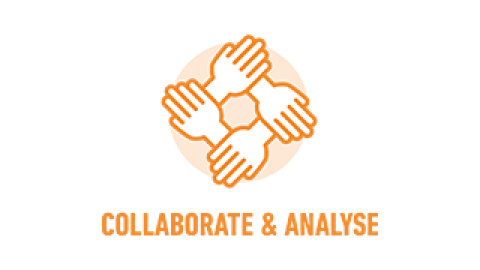
Collaborate & Analyse
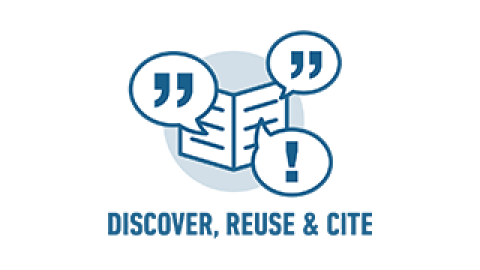
Discover, Reuse & Cite
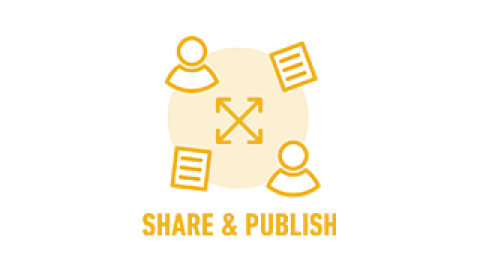
Share & Publish
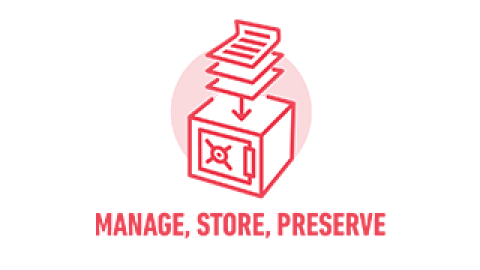